The release of OpenAI’s GPT-2, GPT-3, and GPT-4 followed a similar trajectory: Each iteration was trained in larger, more powerful data centers using increasingly vast datasets. These models outperformed their predecessors so dramatically that many believed scaling alone would drive continued progress. But that may no longer be the case.
AI stagnation concerns. More experts, including OpenAI co-founder Ilya Sutskever, argue that the days of merely adding GPUs and data to improve generative AI models are ending. OpenAI has delayed its next model, Orion, because it doesn’t represent a significant breakthrough. Similarly, Google and Anthropic are also facing slower advances in their developments.
Investment outpaces profits. While tech companies have reaped financial gains from the AI boom—Bloomberg reports they’ve collectively grown by $8 billion over the past two years—revenues directly generated by generative AI systems remain minimal. Despite heavy investments in areas like data centers, the financial returns from these technologies have been largely symbolic.
Enter the sigmoid function. This so-called “stagnation” was inevitable. In technology, progress rarely halts permanently. Instead, breakthroughs often unlock new potential, resembling the sigmoid function or “S-curve.” Progress starts slowly, accelerates sharply, then plateaus—until another innovation spurs renewed growth.
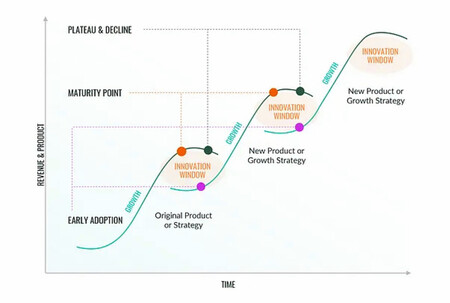
Stagnation to develop. This pattern is evident across fields. Moore’s law in semiconductors, for example, has seemed to falter only to be revitalized by innovations in photolithography. Similarly, commercial aviation was stagnant for years before transitioning from propeller-driven to jet aircraft, a leap that revolutionized the industry. While the pace of innovation in chips and planes has since slowed, efficiency and capabilities continue to improve along a sigmoid-like trajectory.
Turning problems into opportunities. If scaling with GPUs and data is no longer enough, companies must find new methods for developing AI systems. OpenAI has already taken a step with its o1 model, designed to improve reasoning and reduce errors during the inference phase. This shift—from focusing solely on training to optimizing post-training processes—may define the next phase of AI evolution.
A moment to recalibrate. Perhaps this pause is exactly what the fast-moving world of generative AI models needs. With systems already excelling in specific domains—programming being a prime example—it’s an opportunity to explore new development pathways.
Prioritizing safety and regulation. This lull also offers a chance to address safety and regulation, areas that have often been neglected or mishandled. Governments, for instance, must establish clear rules reflecting current AI capabilities rather than speculating on future advancements. The EU AI Act is a promising first step, though its premature introduction highlights the need for adaptability as AI systems evolve. Ensuring AI models are safer and less threatening during this pause could pave the way for responsible innovation.
Image | Tara Winstead
Related | AI Memory Comes at a Cost: Gemini Now Remembers Things as Long as You Pay
View 0 comments